category
大型语言模型(LLM)彻底改变了人工智能领域,创造了与数字世界互动的全新方式。虽然它们提供了一个很好的通用解决方案,但通常必须对它们进行调整以支持特定的域和任务。
人工智能编码助理,或称代码LLM,已经成为帮助实现这一目标的一个领域。到2025年,80%的产品开发生命周期将使用生成性人工智能代码生成,开发人员将充当后端和前端组件及集成的验证器和协调人。您可以为代码任务调整LLM,为开发人员简化工作流程,并降低新手程序员的门槛。代码LLM不仅可以生成代码,还可以填充缺失的代码、添加文档,并提供解决难题的技巧。
这篇文章解释了如何部署端到端代码LLM,包括特定的提示准则、优化技术和客户端服务器部署。我们使用NVIDIA Triton推理服务器,并使用NVIDIA TensorRT LLM进行优化,这是一个用于编译和优化推理LLM的综合库。TensorRT-LLM集成了许多高级优化,同时为定义和构建新模型提供了直观的Python API。
TensorRT LLM开源库加速了NVIDIA GPU上最新LLM的推理性能。它被用作NVIDIA NeMo中LLM推理的优化骨干,这是一个端到端的框架,用于构建、定制生成人工智能应用程序并将其部署到生产中。NeMo框架提供了完整的容器,包括TensorRT LLM和NVIDIA Triton推理服务器,用于生成人工智能部署。它还包含在NVIDIA AI Enterprise中,这是一个生产级、安全且受支持的软件平台,可简化采用过程。
What is AI-assisted coding?
程序员花了很多时间寻找常见问题的解决方法,或者浏览在线论坛以获得更快的代码编写方法。人工智能代码助理背后的关键思想是将程序员需要的信息放在他们正在编写的代码旁边。该工具跟踪程序员正在处理的文件中的代码和注释,以及它链接到的或在同一项目中编辑过的其他文件。它将所有这些文本作为提示发送到LLM。然后,它预测程序员试图做什么,并建议代码来完成它。
Deploying your own AI coding assistant
要部署自己的人工智能编码助手,您需要深度学习推理和LLM的基本知识,以及:
- Hugging Face registered user access and general familiarity with the Transformers library
- Python
- NVIDIA TensorRT-LLM optimization library
- NVIDIA Triton with TensorRT-LLM backend
本教程使用StarCoder,这是一个在The Stack(v1.2)的80多种编程语言上训练的15.5B参数LLM。StarCoder的基本模型是在来自80多种编程语言、GitHub问题、Git委员会和Jupyter笔记本的1万亿个代币上进行训练的。StarCoder在此基础上使用了另一个35B Python令牌进行了微调,从而产生了StarCoder模型。
给定解决特定问题的代码请求,StarCoder可以从头开始生成代码。它还支持中间填充(FIM)或填充,使模型能够生成以插入点周围的前缀和后缀代码为条件的代码(例如,单行填充、返回类型预测和文档字符串生成)。
Prompts for code LLMs
This section features the optimization process. StarCoder was trained on code repositories from the public domain, so it can generate snippets in various languages. A code-based prompt for LLM is a prompt that asks the LLM to generate code in a specific language. The ideal prompt should be specific and clear, providing enough information for the LLM to generate a correct answer.
For example, you could issue a generic prompt such as:
Write a function that computes the square root.
Specifying the programming language and output will yield better results. For example:
Write Python code to compute the square root and print the result.
Prompt engineering for code LLMs
Prompt engineering consists of providing code blocks, single lines of code, or developer comments in order to improve coding suggestions for specific use cases. There are several guidelines to keep in mind with specific prompt engineering for code LLMs, including snippeting and example outputs.
Snippeting
In general, LLMs are limited in the size of their context window. This is especially relevant for code LLMs, as source code often consists of multiple files and long text, often not fitting within LLM context windows. Therefore, prompt engineering for code LLMs requires additional preparation for source code prior to prompting. This can include splitting files into smaller code snippets, choosing the most representative code samples, and using prompt optimization techniques to further decrease the number of input tokens.
Adding example outputs
Prompts that include example outputs and import instructions will yield the best results.
You’ll get average results with a prompt such as:
Write a function for calculating average water use per household.
Adding an example will yield much better results:
Write a function for calculating average water use per household.
Example output ["Smith", 20, "Lincoln", 30, "Simpson", 1500]
And your results will be even better by adding an example and specifying import instructions:
Write a function for calculating average water use per household.
Example input [['Last Name', 'Dishwasher', 'Shower', 'Yard'],
['Smith', 39, 52, 5],
['Lincoln', 25, 77, 18],
['Simpson', 28, 20, 0]]
Example output ["Smith", 32, "Lincoln", 40, "Simpson", 16]
Experiment
Use different prompts and update with finer details at each step to improve output. For example:
Write a function for calculating average water use per household.
Write a function for calculating average water use per household. Add penalties for dishwasher time.
Write a Python function for calculating average water use per household. Add penalties for dishwasher time. Input is family name, address, and number of gallons per specific use.
Overview of flow
To optimize an LLM with TensorRT-LLM, you must understand its architecture and identify which common base architecture it most closely resembles. StarCoder uses the GPT architecture, so this tutorial is based on the NVIDIA/TensorRT-LLM GPT example.
Use the converter and build scripts in this directory to compile StarCoder and prepare it for hardware acceleration. Note that the tokenizer is not handled directly by TensorRT-LLM. However, it is necessary to be able to classify it within a defined tokenizer family for runtime and for setting preprocessing and postprocessing steps in Triton.
Set up and build TensorRT-LLM
Start by cloning and building the TensorRT-LLM library. The easiest way to build TensorRT-LLM and retrieve all its dependencies is to use the included Dockerfile. These commands pull a base container and install all the dependencies needed for TensorRT-LLM inside the container. It then builds and installs TensorRT-LLM itself inside the container.
Retrieve model weights
Download the StarCoder model from Hugging Face and place it in the /examples
directory.
Convert model weights format
Before compilation, convert the model weights format from Hugging Face Transformer to FasterTransformer.
Compile the model
The next step is to compile the model into a TensorRT engine, using the converted weights from the previous step. Compilation has many options, such as precision and selecting which optimization features to enable (in-flight batching and KV caching, for example).
Run the model
TensorRT-LLM includes a highly optimized C++ runtime for executing built LLM engines and managing processes like sampling tokens from the model output, managing the KV cache, and batching requests together. You can use that runtime directly to execute the model locally.
You can experiment by using a more complex prompt, including the definition of the function name, parameters, and output.
Deploy the model on NVIDIA Triton
We recommend using NVIDIA Triton Inference Server, an open-source platform that streamlines and accelerates the deployment of AI inference workloads to create a production-ready deployment of your LLM. This will help reduce setup and deployment time. The Triton Inference Server backend for TensorRT-LLM leverages the TensorRT-LLM C++ runtime for rapid inference execution and includes techniques like in-flight batching and paged KV caching. You can access Triton Inference Server with the TensorRT-LLM backend as a prebuilt container through the NVIDIA NGC catalog.
First, create a model repository so Triton can read the model and any associated metadata. The tensorrtllm_backend
repository includes the skeleton of an appropriate model repository under all_models/inflight_batcher_llm/
. In that directory are the following subfolders that hold artifacts for different parts of the model execution process:
/preprocessing
and/postprocessing
: Contain scripts for the Triton backend for Python for tokenizing the text inputs and detokenizing the model outputs to convert between strings and the token IDs on which the model operates./tensorrt_llm
: A subfolder for storing the model engine that you previously compiled./ensemble
: Defines a model ensemble that links the previous three components together and tells Triton how to flow data through them.
Pay close attention to the parameter kv_cache_free_gpu_mem_fraction, which handles memory allocation for the KV cache during inference. This parameter is set with a number between 0 and 1, and allocates GPU memory accordingly. Setting this to 1 will fill the GPU memory capacity to the maximum.
Setting this parameter is especially important when deploying large LLMs or running multiple instances of TensorRT-LLM on a single GPU. Note that the default value is 0.85. In the case of StarCoder for this tutorial, we used a value of 0.2 to avoid filling GPU memory.
With the KV cache, intermediate data is cached and reused, avoiding recomputation. Instead of recomputing full key and value matrices in every iteration, intermediate feature maps are cached and reused in the next iteration. This reduces the computational complexity of the decoding mechanism. The memory consumption of the KV cache can easily fill terabytes of data for production-level LLMs.
Another important parameter to note is the tokenizer. You need to specify the appropriate tokenizer, as well as which category type it best fits into, to define the preprocessing and postprocessing steps required for Triton. StarCoder uses code as input, rather than sentences, so it has unique tokens and separators.
As such, it is necessary to use the specific StarCoder tokenizer by setting the tokenizer_type
to auto and the tokenizer_dir
to /all_models/startcoder
in the configuration files. You can set this by calling fill_template.py
or editing the config.pbtxt
file directly.
Launch Triton
To launch Triton, first spin up the Docker container, then launch the server script specifying the numbers of GPUs the model was built for by setting the world_size
parameter.
Send requests
To send requests to and interact with the running server, you can use one of the Triton client libraries or send HTTP requests to the generated endpoint.
To get started with a simple request, use the following curl command to send HTTP requests to the generated endpoint:
You can also use the more fully featured client script to interact with the running server.
Running the command above will generate the code below. The resulting plot is shown in Figure 1.
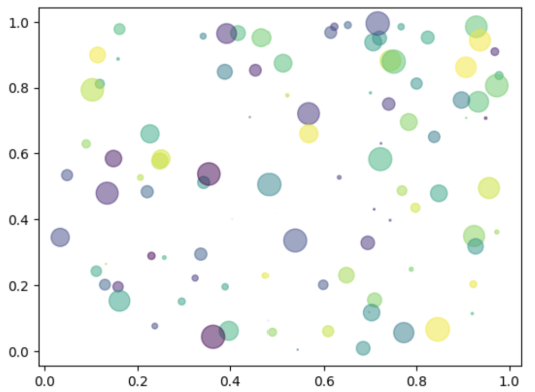
Get started
With baseline support for many popular LLM architectures, NVIDIA TensorRT-LLM and NVIDIA Triton make it easy to deploy, optimize, and run a variety of code LLMs. To get started, download and set up the NVIDIA/TensorRT-LLM open-source library on GitHub, and experiment with the different example LLMs. You can also download the StarCoder model and follow the steps in this post for hands-on experience.
Related resources
- DLI course: Deploying a Model for Inference at Production Scale
- GTC session: Deploying, Optimizing, and Benchmarking Large Language Models With Triton Inference Server
- GTC session: Accelerated LLM Model Alignment and Deployment in NeMo, TensorRT-LLM, and Triton Inference Server
- SDK: TensorRT-ONNX Runtime
- SDK: TensorFlow-TensorRT
- SDK: TensorRT
- 登录 发表评论
- 25 次浏览
最新内容
- 6 days 12 hours ago
- 1 week ago
- 1 week 3 days ago
- 1 week 4 days ago
- 1 week 5 days ago
- 1 week 5 days ago
- 1 week 5 days ago
- 1 week 5 days ago
- 1 week 6 days ago
- 2 weeks 5 days ago